Causal Incrementality with Marketing Mix Modeling
- Gabriel Mohanna
- Aug 21, 2024
- 2 min read
Updated: Oct 8, 2024
Marketing Mix Modeling (MMM) is a powerful tool for understanding the effectiveness of marketing strategies. However, a common pitfall in MMM is the confusion between correlation and causation. Traditionally, randomized control trials (RCTs) and Geo testing are used to correct this, but they’re costly and time-consuming. At MMM Labs, the process starts with better models - models that provide reliable estimates before you even begin experimenting.
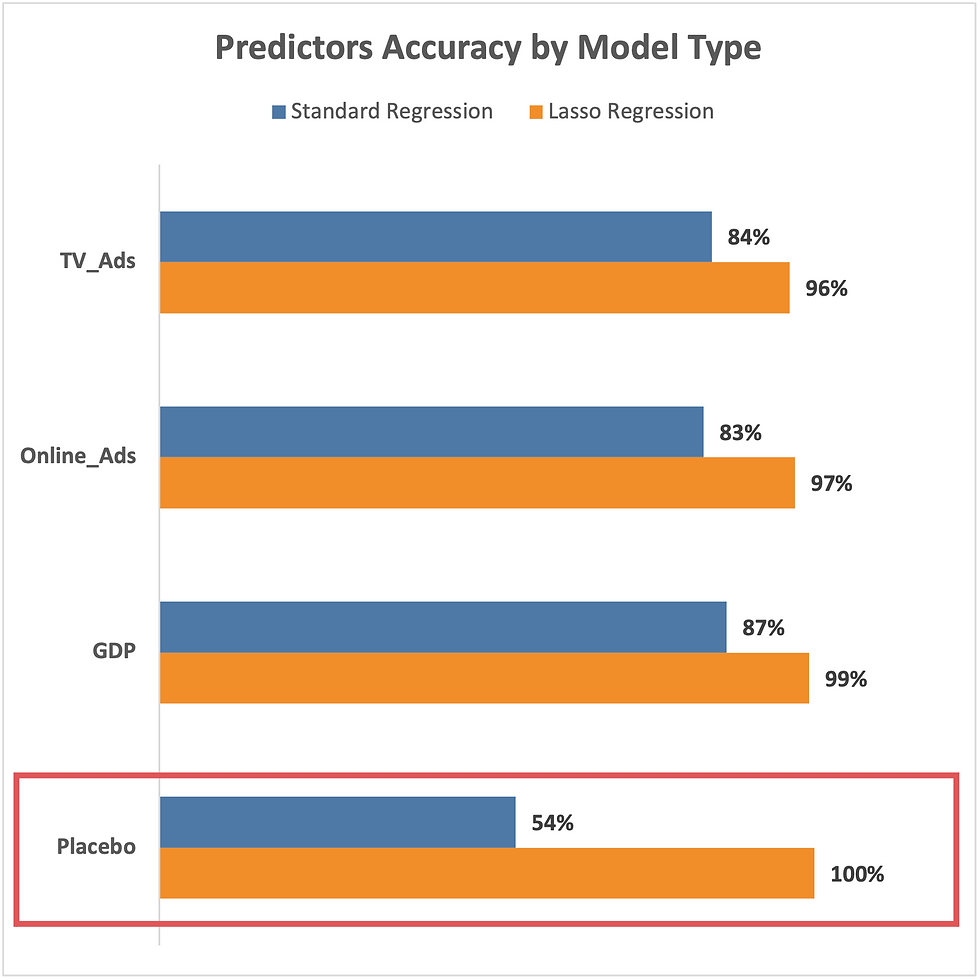
How We Address Causality with MMM: (Simulated Incrementality)
At MMM Labs, we conducted an experiment to demonstrate the importance of distinguishing between correlation and true causality. We simulated a dataset with actual incremental marketing drivers and included a placebo variable - something that is correlated with sales but has no impact (see the code on our GitHub page).
We then ran two types of analyses: a standard regression (OLS) and a more advanced technique called Lasso regression.
Standard Regression (OLS): This is the most common MMM approach. It's easy to start from. But mistakenly attribute sales lift to irrelevant factors, confusing correlation with causation.
Lasso Regression: This is an advanced Machine Learning (ML) technique. It improves the estimation process by filtering out irrelevant factors, focusing only on the true drivers of sales, and enhancing the model's predictive power.
The Results
Our quick experiment showed that the standard regression mistakenly attributed an incremental sales lift (46%) to our placebo variable, which had no real impact on sales. In contrast, Lasso regression (just one of many tools at MMM Labs), was able to filter out the noise and focus on the true drivers of sales.
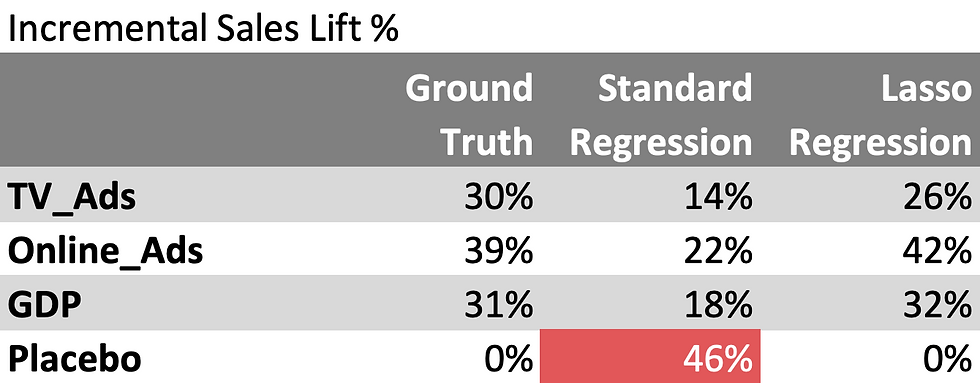
Why It Matters?
This distinction has significant implications. Misinterpreting your data by using the wrong model can lead to costly mistakes, diverting resources to ineffective strategies. While experiments and Geo tests are essential components of validating marketing effectiveness, starting with a model that provides accurate, causal insights ensures that your subsequent testing is both effective and efficient. By leveraging advanced models, you can better allocate your marketing spend and achieve more reliable results from your tests.
Take Action
At MMM Labs, we ensure you make the right decisions and help you get it right from the start. Curious to see how Meta's Robyn compares to Google's Lightweight MMM or Meridian? Book a demo with us at MMM Labs, and let’s explore how our advanced models can help you make data-driven decisions that reveal true causality incrementality, setting the stage for more targeted experiments and tests.